Navigating the Ethical Frontiers of AI in Healthcare
This summary was automatically produced by experimental artificial intelligence (AI)-powered technology. It may contain inaccuracies or omissions; see the full presentation before relying on this information for medical decision-making. If you see a problem, please report it to us here.
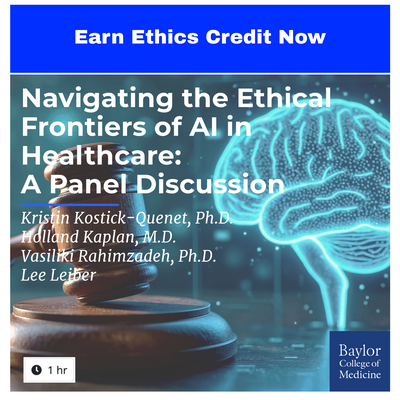
Introduction
This document presents excerpts from a panel discussion titled "Navigating the Ethical Frontiers of AI in Healthcare," the sixth session of an eight-week virtual series on AI in healthcare. The discussion brings together four experts: Holland Kaplan, M.D.
(Assistant Professor of Clinical Ethics and General Internal Medicine), Kristin Kostick-Quenet, Ph.D. (Assistant Professor, Center for Medical Ethics and Health Policy), Vasiliki Rahimzadeh, Ph.D. (Assistant Professor in the Center for Medical Ethics and Health Policy), and Lee Leiber (Chief Information Officer at Baylor College of Medicine). The central theme revolves around the increasing integration of AI in clinical care and the crucial ethical challenges that arise from its use.
Foundational Concepts in AI Ethics
Dr. Kostick-Quenet provides a foundational overview of AI and its ethical implications. She highlights that while a complete consensus on addressing AI ethics is still evolving, there is a growing agreement on the central ethical challenges.
- Defining AI: AI broadly encompasses techniques where computers are programmed to mimic human intelligence and analytical reasoning.
- Types of AI: The discussion touches upon different types of AI:
- Machine Learning: A type of AI that learns from experience rather than explicit step-by-step instructions. Its dynamic nature poses challenges for regulation.
- Deep Learning: Inspired by the human brain, involving artificial neural networks for parallel distributed processing.
- Generative AI: Revolutionary as it can create new and original content (text, images, music, code) by learning from vast datasets, particularly natural language data and large language models. This mimics human creativity.
- The Core Question of AI Ethics: How to appraise AI systems, their users, and the context of their use to ensure safe and responsible performance. Key considerations include accuracy, trustability, and fairness.
- Ethical Considerations Across the AI Lifecycle: Ethical considerations are relevant at every stage, from development to validation, testing, and implementation.
- Development: Crucial aspects include data quality (high quality, well-curated, representative of end-users), ethical data collection (consent, compensation, credit for data use), and data security (protection against re-identification and misuse of open-source data).
- Validation and Testing: Algorithmic bias is a major concern. Training data from non-representative populations can lead to inaccurate and irrelevant outputs, exacerbating health disparities. Thorough testing across broad and diverse cohorts (sociodemographic, ethnicity, clinical characteristics, geography, treatment settings) is essential to ensure validity and generalizability. Lack of such testing can compromise patient safety.
- Implementation: Key questions involve the intended use of the AI system (on-label vs. off-label use), the potential impact on diverse populations not represented in the training data, and the appropriate level of reliance on the system. There are no established evidence-based best practices for AI implementation, making it crucial to find a balance between over-reliance (leading to suboptimal decisions if the AI is wrong) and under-reliance (missing potentially beneficial insights).
- Transparency as a Foundational Principle: Transparency is highlighted as a core feature enabling informed appraisal of AI systems and underpinning other ethical considerations. Developers have a responsibility to convey sufficient information about a system's performance capacities to end-users to facilitate critical evaluation and responsible integration into practice.
Addressing Specific Ethical Dilemmas
The panel discussion then delves into specific case studies to illustrate these ethical challenges in healthcare settings.
- Algorithmic Bias: A case involving an AI-driven dermatology application misdiagnosing melanoma in darker-skinned patients due to training data predominantly featuring fair-skinned individuals highlights the dangers of algorithmic bias. Strategies to mitigate this include interrogating and balancing data sets (though procuring new data can be challenging) and carefully considering whether to include or exclude protected characteristics as variables. The importance of recognizing and addressing bias already present in existing data (as seen with GFR calculator adjustments) is emphasized. Testing models with retrospective data before prospective implementation is suggested as an initial step when acquiring models developed elsewhere.
- Transparency and Explainability: A scenario where an AI tool recommends a less aggressive treatment plan for a diabetes patient without providing a rationale raises concerns about transparency and explainability. While the importance of these is acknowledged, the discussion explores the level of detail necessary for different stakeholders. There's a suggestion that the deep explainability might be more critical for developers, while for clinicians and patients, the focus might shift towards clear disclosure about when and how AI is being used in their care. The challenge of effectively communicating AI's role to patients, especially given potential misconceptions, is noted, emphasizing the need for patient education alongside disclosure. Different levels of transparency and explainability may be required depending on the AI's use case (e.g., risk calculators based on quantitative data versus systems incorporating qualitative patient preferences).
- Accountability and Responsibility: A case where an AI system fails to detect early-stage pneumonia, leading to delayed treatment, brings up the complex issue of accountability. With distributed responsibility among radiologists, hospital administration, and AI developers, determining liability is challenging. Existing accountability mechanisms for medical technologies are challenged by the dynamic nature of AI. The discussion considers the professional responsibility of physicians, medical liability, and the potential for enterprise liability, where hospitals or health systems deploying AI technologies bear greater accountability due to their comprehensive understanding of the technology and its implementation environment. This could incentivize greater scrutiny of AI adoption. The importance of keeping the "human in the loop" during development and implementation is stressed, ensuring that clinical expertise remains integral. However, the emergence of autonomous AI agents ("human on the loop") presents new challenges to this paradigm.
- Impact on the Patient-Physician Relationship: The use of AI to generate automated responses to patient messages raises concerns about maintaining the humanistic aspects of care. A scenario where a generic AI response distresses a patient highlights the potential for eroding trust. While AI might augment or even seemingly replicate certain aspects of the relationship (e.g., AI therapy, empathetic chatbot responses), the panel suggests that complete replacement is neither practical nor desirable soon. Instead, AI tools could potentially serve as a humanistic accountability mechanism, helping clinicians refine their communication and empathy. The importance of distinguishing between appropriate and inappropriate delegation of aspects of the patient-physician relationship to AI is emphasized.
Governance of AI in Healthcare
Lee Leiber addresses the critical aspect of governance for the increasing influx of AI solutions in healthcare. He cautions against sharing sensitive patient information (PHI) with unsecured AI platforms, highlighting HIPAA violations.
- Baylor College of Medicine's Initiatives: The institution has published institutional guidelines for the use of generative AI, developed by a cross-functional working group. Key tenets include:
- Never sharing PHI, PII, or other confidential information with AI platforms without a Business Associate Agreement.
- Maintaining "human in the loop" by validating AI-generated information.
- Disclosing the use of AI tools where appropriate.
- Adhering to existing Baylor policies governing data use and protection.
- Shift Towards Governance: Baylor is transitioning from guidance to formal governance to address the increasing velocity of AI adoption with limited team resources, aiming to mitigate potential institutional risks (including past small data breaches). This includes building centralized oversight and collegewide governance.
- Review Process for New Tools: Governance is in place for the purchase of new AI tools, requiring review from compliance, IT, and information security.
- Health Information Technology Integration and Innovation Committee (HIT): This committee, under the clinical mission, vets new AI and other technology solutions for clinical practice, requiring final approval by the board of governors. Its goals include aligning with Baylor's mission, ensuring positive ROI, and upholding the brand promise. Ambient listening technology is an example of a current focus.
- Key Takeaways on Governance: The pace of AI innovation is likely to exceed the pace of governance development across all industries. Familiarity with institutional data protection policies is crucial, and the compliance and information security teams are available for guidance.
Conclusion
The panel discussion underscores the multifaceted ethical challenges and opportunities presented by the increasing integration of AI in healthcare. It highlights the importance of addressing algorithmic bias, ensuring transparency and appropriate explainability, navigating complex accountability issues, thoughtfully considering the impact on the patient-physician relationship, and establishing robust governance frameworks to guide the ethical and safe adoption of AI in clinical practice.
Artificial intelligence (AI) was used to transcribe the presentation’s contents and create a summary of the information contained in the presentation. This is an experimental process, and while we strive for accuracy, AI-generated content may not always be perfect and could contain errors. This summary has not been reviewed by the presenter to guarantee completeness or correctness of the content, so it should not be used for medical decision-making without reviewing the original presentation.
If you have feedback, questions, or concerns please contact us here.